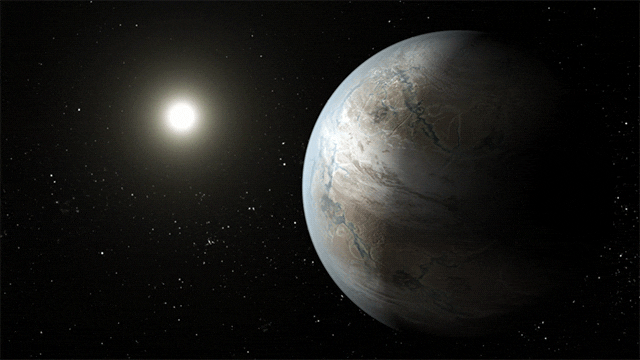
태양과 같은 별(왼쪽)과 별의 거주 가능 영역에서 궤도에 있는 지구보다 약 60% 더 큰 암석 행성에 대한 예술가의 개념. 중력 마이크로 렌즈는 행성 자체가 너무 불투명하여 볼 수 없을지라도 그러한 행성계를 감지하고 궤도 질량과 거리를 결정할 수 있는 능력을 가지고 있습니다. 이미지 크레디트: NASA Ames / JPL-Caltech / T. Pyle
머신 러닝 알고리즘은 마이크로렌싱의 해석에 대한 수학적 이론의 문제를 지적합니다.
실제 천문 관측으로 훈련된 인공 지능(AI) 시스템은 새로운 초신성을 찾고, 새로운 유형의 은하를 식별하고, 거대한 별 병합을 발견하기 위해 방대한 양의 데이터를 필터링하는 데 천문학자를 우회하여 세계에서 새로운 발견의 비율을 높입니다. 나는 과학을 제안합니다.
하지만 머신 러닝이라고 하는 인공 지능은 더 깊은 것을 드러낼 수 있습니다.[{” attribute=””>University of California, Berkeley, astronomers found: unsuspected connections hidden in the complex mathematics arising from general relativity — in particular, how that theory is applied to finding new planets around other stars.
In a paper published on May 23, 2022, in the journal Nature Astronomy, the researchers describe how an AI algorithm developed to more quickly detect exoplanets when such planetary systems pass in front of a background star and briefly brighten it — a process known as gravitational microlensing — revealed that the decades-old theories now used to explain these observations are woefully incomplete.
In 1936, Albert Einstein himself used his new theory of general relativity to show how the light from a distant star can be bent by the gravity of a foreground star, not only brightening it as seen from Earth, but often splitting it into several points of light or distorting it into a ring, now called an Einstein ring. This is similar to the way a hand lens can focus and intensify light from the sun.
But when the foreground object is a star with a planet, the brightening over time — the light curve — is more complicated. What’s more, there are often multiple planetary orbits that can explain a given light curve equally well — so called degeneracies. That’s where humans simplified the math and missed the bigger picture.
Seen from Earth (left), a planetary system moving in front of a background star (source, right) distorts the light from that star, making it brighten as much as 10 or 100 times. Because both the star and exoplanet in the system bend the light from the background star, the masses and orbital parameters of the system can be ambiguous. An AI algorithm developed by UC Berkeley astronomers got around that problem, but it also pointed out errors in how astronomers have been interpreting the mathematics of gravitational microlensing. Credit: Diagram courtesy of Research Gate
The AI algorithm, however, pointed to a mathematical way to unify the two major kinds of degeneracy in interpreting what telescopes detect during microlensing, showing that the two “theories” are really special cases of a broader theory that, the researchers admit, is likely still incomplete.
“A machine learning inference algorithm we previously developed led us to discover something new and fundamental about the equations that govern the general relativistic effect of light- bending by two massive bodies,” Joshua Bloom wrote in a blog post last year when he uploaded the paper to a preprint server, arXiv. Bloom is a UC Berkeley professor of astronomy and chair of the department.
He compared the discovery by UC Berkeley graduate student Keming Zhang to connections that Google’s AI team, DeepMind, recently made between two different areas of mathematics. Taken together, these examples show that AI systems can reveal fundamental associations that humans miss.
“I argue that they constitute one of the first, if not the first time that AI has been used to directly yield new theoretical insight in math and astronomy,” Bloom said. “Just as Steve Jobs suggested computers could be the bicycles of the mind, we’ve been seeking an AI framework to serve as an intellectual rocket ship for scientists.”
“This is kind of a milestone in AI and machine learning,” emphasized co-author Scott Gaudi, a professor of astronomy at The Ohio State University and one of the pioneers of using gravitational microlensing to discover exoplanets. “Keming’s machine learning algorithm uncovered this degeneracy that had been missed by experts in the field toiling with data for decades. This is suggestive of how research is going to go in the future when it is aided by machine learning, which is really exciting.”
Discovering exoplanets with microlensing
More than 5,000 exoplanets, or extrasolar planets, have been discovered around stars in the Milky Way, though few have actually been seen through a telescope — they are too dim. Most have been detected because they create a Doppler wobble in the motions of their host stars or because they slightly dim the light from the host star when they cross in front of it — transits that were the focus of NASA’s Kepler mission. Little more than 100 have been discovered by a third technique, microlensing.
This infographic explains the light curve astronomers detect when viewing a microlensing event, and the signature of an exoplanet: an additional uptick in brightness when the exoplanet lenses the background star. Credit: NASA, ESA, and K. Sahu (STScI)
One of the main goals of NASA’s Nancy Grace Roman Space Telescope, scheduled to launch by 2027, is to discover thousands more exoplanets via microlensing. The technique has an advantage over the Doppler and transit techniques in that it can detect lower-mass planets, including those the size of Earth, that are far from their stars, at a distance equivalent to that of Jupiter or Saturn in our solar system.
Bloom, Zhang and their colleagues set out two years ago to develop an AI algorithm to analyze microlensing data faster to determine the stellar and planetary masses of these planetary systems and the distances the planets are orbiting from their stars. Such an algorithm would speed analysis of the likely hundreds of thousands of events the Roman telescope will detect in order to find the 1% or fewer that are caused by exoplanetary systems.
One problem astronomers encounter, however, is that the observed signal can be ambiguous. When a lone foreground star passes in front of a background star, the brightness of the background stars rises smoothly to a peak and then drops symmetrically to its original brightness. It’s easy to understand mathematically and observationally.
But if the foreground star has a planet, the planet creates a separate brightness peak within the peak caused by the star. When trying to reconstruct the orbital configuration of the exoplanet that produced the signal, general relativity often allows two or more so-called degenerate solutions, all of which can explain the observations.
To date, astronomers have generally dealt with these degeneracies in simplistic and artificially distinct ways, Gaudi said. If the distant starlight passes close to the star, the observations could be interpreted either as a wide or a close orbit for the planet — an ambiguity astronomers can often resolve with other data. A second type of degeneracy occurs when the background starlight passes close to the planet. In this case, however, the two different solutions for the planetary orbit are generally only slightly different.
According to Gaudi, these two simplifications of two-body gravitational microlensing are usually sufficient to determine the true masses and orbital distances. In fact, in a paper published last year, Zhang, Bloom, Gaudi, and two other UC Berkeley co-authors, astronomy professor Jessica Lu and graduate student Casey Lam, described a new AI algorithm that does not rely on knowledge of these interpretations at all. The algorithm greatly accelerates analysis of microlensing observations, providing results in milliseconds, rather than days, and drastically reducing the computer crunching.
Zhang then tested the new AI algorithm on microlensing light curves from hundreds of possible orbital configurations of star and exoplanet and discovered something unusual: There were other ambiguities that the two interpretations did not account for. He concluded that the commonly used interpretations of microlensing were, in fact, just special cases of a broader theory that explains the full variety of ambiguities in microlensing events.
“The two previous theories of degeneracy deal with cases where the background star appears to pass close to the foreground star or the foreground planet,” Zhang said. “The AI algorithm showed us hundreds of examples from not only these two cases, but also situations where the star doesn’t pass close to either the star or planet and cannot be explained by either previous theory. That was key to us proposing the new unifying theory.”
Gaudi was skeptical, at first, but came around after Zhang produced many examples where the previous two theories did not fit observations and the new theory did. Zhang actually looked at the data from two dozen previous papers that reported the discovery of exoplanets through microlensing and found that, in all cases, the new theory fit the data better than the previous theories.
“People were seeing these microlensing events, which actually were exhibiting this new degeneracy but just didn’t realize it,” Gaudi said. “It was really just the machine learning looking at thousands of events where it became impossible to miss.”
Zhang and Gaudi have submitted a new paper that rigorously describes the new mathematics based on general relativity and explores the theory in microlensing situations where more than one exoplanet orbits a star.
The new theory technically makes interpretation of microlensing observations more ambiguous, since there are more degenerate solutions to describe the observations. But the theory also demonstrates clearly that observing the same microlensing event from two perspectives — from Earth and from the orbit of the Roman Space Telescope, for example — will make it easier to settle on the correct orbits and masses. That is what astronomers currently plan to do, Gaudi said.
“The AI suggested a way to look at the lens equation in a new light and uncover something really deep about the mathematics of it,” said Bloom. “AI is sort of emerging as not just this kind of blunt tool that’s in our toolbox, but as something that’s actually quite clever. Alongside an expert like Keming, the two were able to do something pretty fundamental.”
Reference: “A ubiquitous unifying degeneracy in two-body microlensing systems” by Keming Zhang, B. Scott Gaudi and Joshua S. Bloom, 23 May 2022, Nature Astronomy.
DOI: 10.1038/s41550-022-01671-6
“요은 베이컨과 알코올에 대한 전문 지식을 가진 닌자입니다. 그의 탐험적인 성격은 다양한 경험을 통해 대중 문화에 대한 깊은 애정과 지식을 얻게 해주었습니다. 그는 자랑스러운 탐험가로서, 새로운 문화와 경험을 적극적으로 탐구하며, 대중 문화에 대한 그의 열정은 그의 작품 속에서도 느낄 수 있습니다.”